In 2024, big data continues to be a cornerstone of innovation and decision-making across industries. From powering artificial intelligence (AI) systems to enabling real-time analytics, big data is driving transformative change. However, the rapid evolution of data generation, processing, and utilization has introduced new challenges. Here, we delve into the most pressing big data challenges of 2024 and how organizations can navigate them.
1. Data Privacy in the Age of AI
As AI technologies like generative AI and advanced machine learning models become mainstream, they demand vast quantities of data for training. However, this intensifies concerns over data privacy and compliance with evolving regulations such as GDPR, CCPA, and newer frameworks like India’s Digital Personal Data Protection Act (DPDP).
Challenge: Organizations must ensure that sensitive data used to train AI models is anonymized and secure while complying with increasingly stringent global and regional regulations.
Solution: Implement advanced privacy-preserving techniques like federated learning and differential privacy. Regular audits and investing in robust data governance tools can also help maintain compliance.
2. Exponential Growth of Unstructured Data
Unstructured data, such as videos, images, audio, and social media posts, is growing exponentially. By 2024, it’s estimated that unstructured data accounts for over 80% of all enterprise data. However, extracting insights from this type of data remains a formidable challenge.
Challenge: Traditional data management and processing systems struggle to efficiently handle the volume and variety of unstructured data.
Solution: Organizations must adopt advanced technologies like natural language processing (NLP) and computer vision while leveraging scalable storage solutions such as object storage and cloud-native platforms.
3. Real-Time Data Processing and Analytics
The demand for real-time insights has surged, especially in sectors like e-commerce, finance, and healthcare. Businesses now need to process massive data streams from IoT devices, social media, and transactional systems in real-time.
Challenge: Existing data pipelines often face latency issues and struggle to scale with the increasing velocity of data streams.
Solution: Adopting frameworks like Apache Kafka, Flink, or Spark Streaming can help manage real-time data more effectively. Additionally, edge computing is becoming a critical strategy for reducing latency by processing data closer to the source.
4. Data Quality and Integrity
In 2024, the adage “garbage in, garbage out” still holds true. Poor data quality undermines analytics and AI efforts, leading to flawed insights and business decisions.
Challenge: Organizations are overwhelmed by the sheer volume of data, making it harder to identify and rectify inconsistencies, duplicates, and inaccuracies.
Solution: Invest in automated data quality tools that utilize AI to detect anomalies and enforce data cleansing. A proactive approach to data governance, including metadata management and lineage tracking, is also essential.
5. Rising Costs of Data Storage
With data volumes doubling every few years, the cost of storing and managing data has become a significant burden. Enterprises are grappling with the financial and environmental costs of maintaining massive data warehouses and cloud storage systems.
Challenge: Balancing the need for data retention with cost-effective storage solutions is a growing concern.
Solution: Organizations should prioritize data lifecycle management, archiving cold data, and adopting tiered storage systems. Emerging technologies like DNA-based data storage could also offer innovative solutions in the near future.
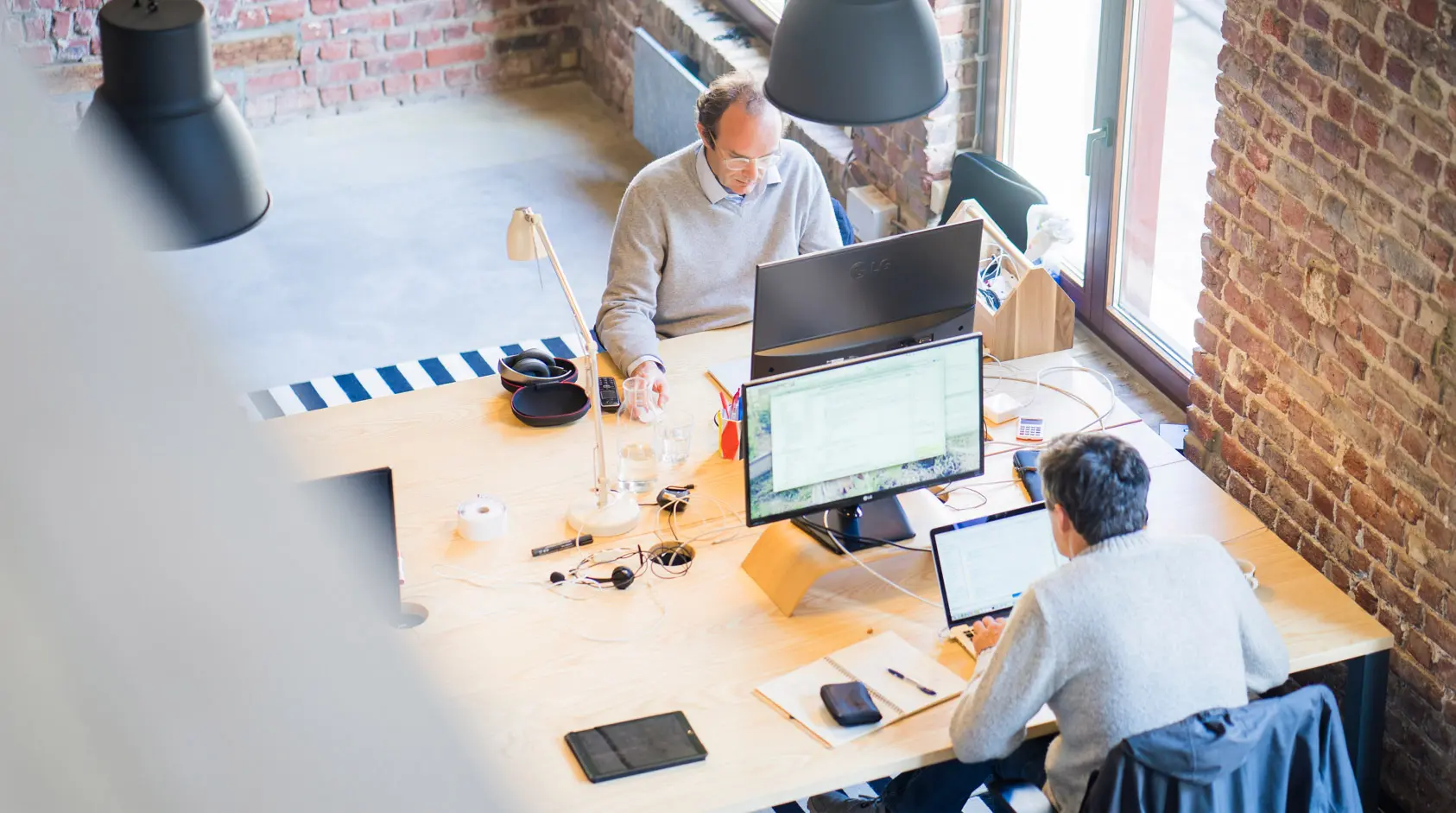
6. Cybersecurity Threats
The value of data makes it a prime target for cybercriminals. From ransomware attacks to data breaches, the risks associated with big data have escalated dramatically.
Challenge: Protecting vast data ecosystems from increasingly sophisticated cyber threats is a daunting task.
Solution: Invest in robust cybersecurity measures such as AI-driven threat detection, zero-trust architectures, and encryption standards. Employee training and adherence to cybersecurity best practices are also crucial.
7. Talent Gap in Big Data Analytics
Despite advancements in automation, the demand for skilled data scientists, analysts, and engineers continues to outstrip supply. This talent gap hinders organizations from fully leveraging their data assets.
Challenge: Attracting, training, and retaining talent in a highly competitive landscape is difficult.
Solution: Organizations can upskill their existing workforce through training programs and leverage citizen data science tools to democratize data analysis. Collaboration with academic institutions and offering competitive compensation packages can also help bridge the gap.
8. Ethical Considerations in Data Use
The rise of AI and predictive analytics has sparked ethical debates about how data is collected, processed, and utilized. Issues like algorithmic bias and lack of transparency in decision-making remain hot topics.
Challenge: Building trust with stakeholders while navigating ethical dilemmas is more critical than ever.
Solution: Develop clear ethical guidelines and ensure that data practices align with societal values. Implement explainable AI (XAI) techniques to foster transparency in algorithms and analytics.
Conclusion
The challenges of big data in 2024 highlight the growing complexity of managing, securing, and extracting value from data. While these hurdles are significant, they also present opportunities for innovation. By adopting cutting-edge technologies, enhancing governance, and fostering a data-driven culture, organizations can not only overcome these challenges but also thrive in the age of big data.
What challenges is your organization facing with big data? Share your thoughts in the comments below!
Not only will a network administrator keep you safe, but you will not have to waste as much time going through unwanted emails. Protect yourself before the inevitable happens.
Comments are closed.