The verification of work done by AI faces several critical challenges. One of the most important is the lack of a clear definition of what exactly should be audited and how. The complexity of the systems and the changing operational environment create additional difficulties in the assessment.
Lack of expert capacity
Human resource problems:
– Demand for qualified AI auditors significantly exceeds supply
– Professionals face dual pressures: in addition to developing their own AI-based practice, they must also learn to audit AI systems
– High demand increases the cost and duration of auditing
Technical challenges
Data integrity and model robustness:
– Assessing the performance and reliability of AI systems is a complex task
– Testing must cover data accuracy and representativeness
– Achieving 100% accuracy is not a realistic expectation
Security considerations
Critical areas:
– Verification of version control and software management
– Testing cyber security and data protection
– Protecting the integrity of systems against unauthorised access
Ethical considerations
Ethical issues in AI auditing:
– Possibility of biased decision making
– Job loss concerns
– Limited human oversight
Future prospects
Need for successful AI quality assurance:
– A thorough understanding of the capabilities and limitations of AI
– Providing reliable and accurate data
– Develop relevant models and algorithms
The key to effective QA is continuous adaptation and regular monitoring of dynamic model updates, in addition to managing stakeholder expectations.
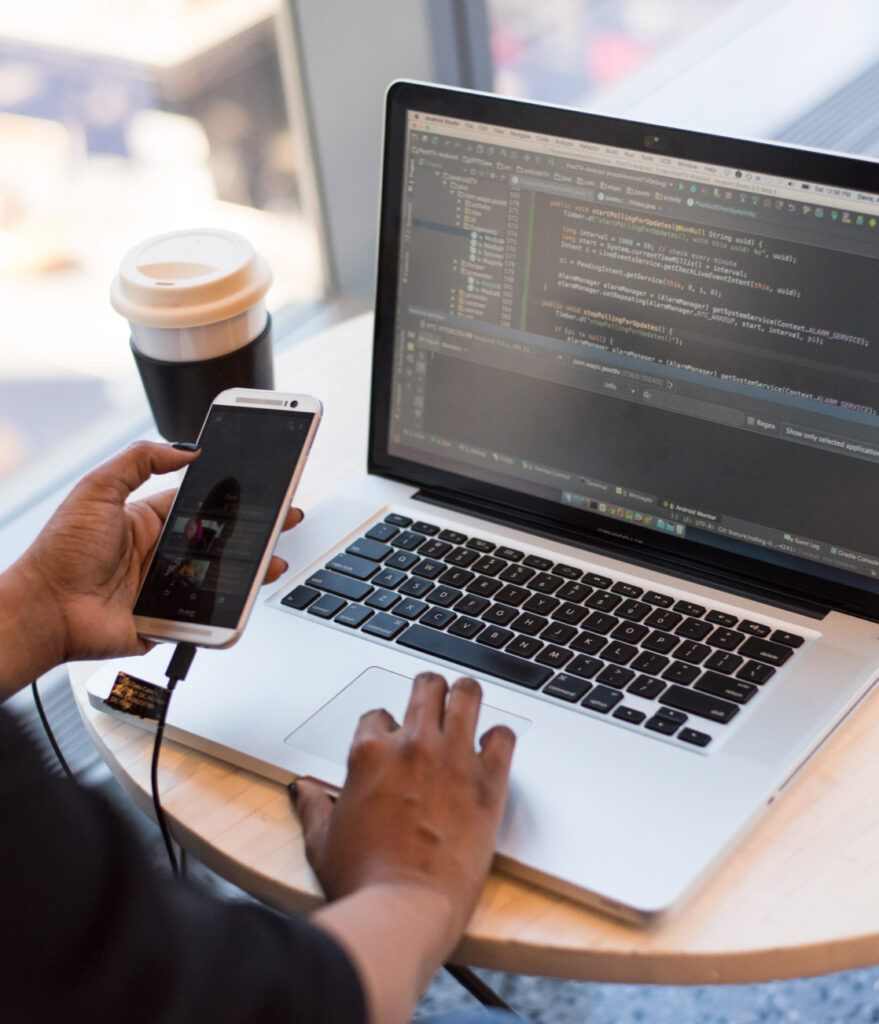